The Personalization guide
What is personalization and how to get started?
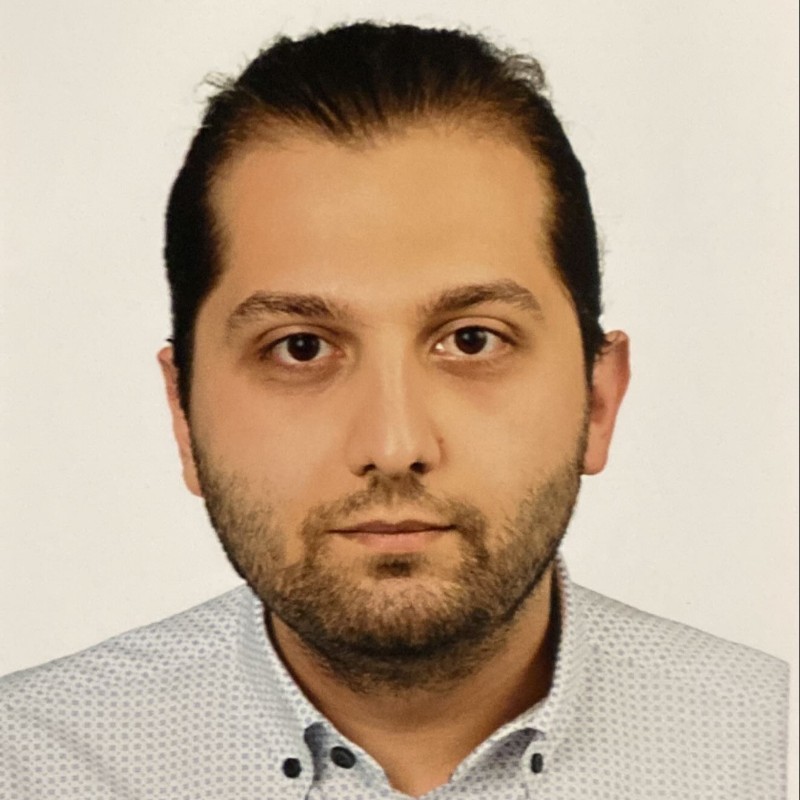
Esat Artug
Updated: February 4, 2025
The Personalization guide
- 1. What is personalization and how to get started?
- 2. Web content personalization
- 3. Extend your reach with omnichannel personalization
- 4. Deliver personalized ecommerce experiences
- 5. Hyper-personalization: The next level of personalized content
- 6. How AI personalization can level up your marketing strategy
- 7. 5 powerful benefits of personalization
In this guide, you’ll learn about the benefits of personalization, different methods for personalizing customer experiences, and how to develop and implement different marketing personalization strategies successfully.
Introduction
Personalization is a vital strategy for increasing user engagement and customer retention in a busy digital world. Today’s personalized content is far more sophisticated than just a name at the top of an email. Customers expect brands to tailor content in real time across channels and devices based on their viewing history, past transactions, and individual preferences.
For most companies, the biggest challenge is scaling personalized content. Creating and managing personalized experiences for each visitor takes time and effort. But with AI-native personalization tools, even small teams can deliver enterprise-level personalization that wins hearts and drives conversion.
That makes now the perfect time to learn more about different personalization strategies and how you can implement them.
What is personalization in marketing?
Personalization is a process of continuously optimizing the customer experience to deliver the right content to the right person at the right time on the right channel.
This means tailoring content to match individual preferences and delivering it when and where the customer needs it.
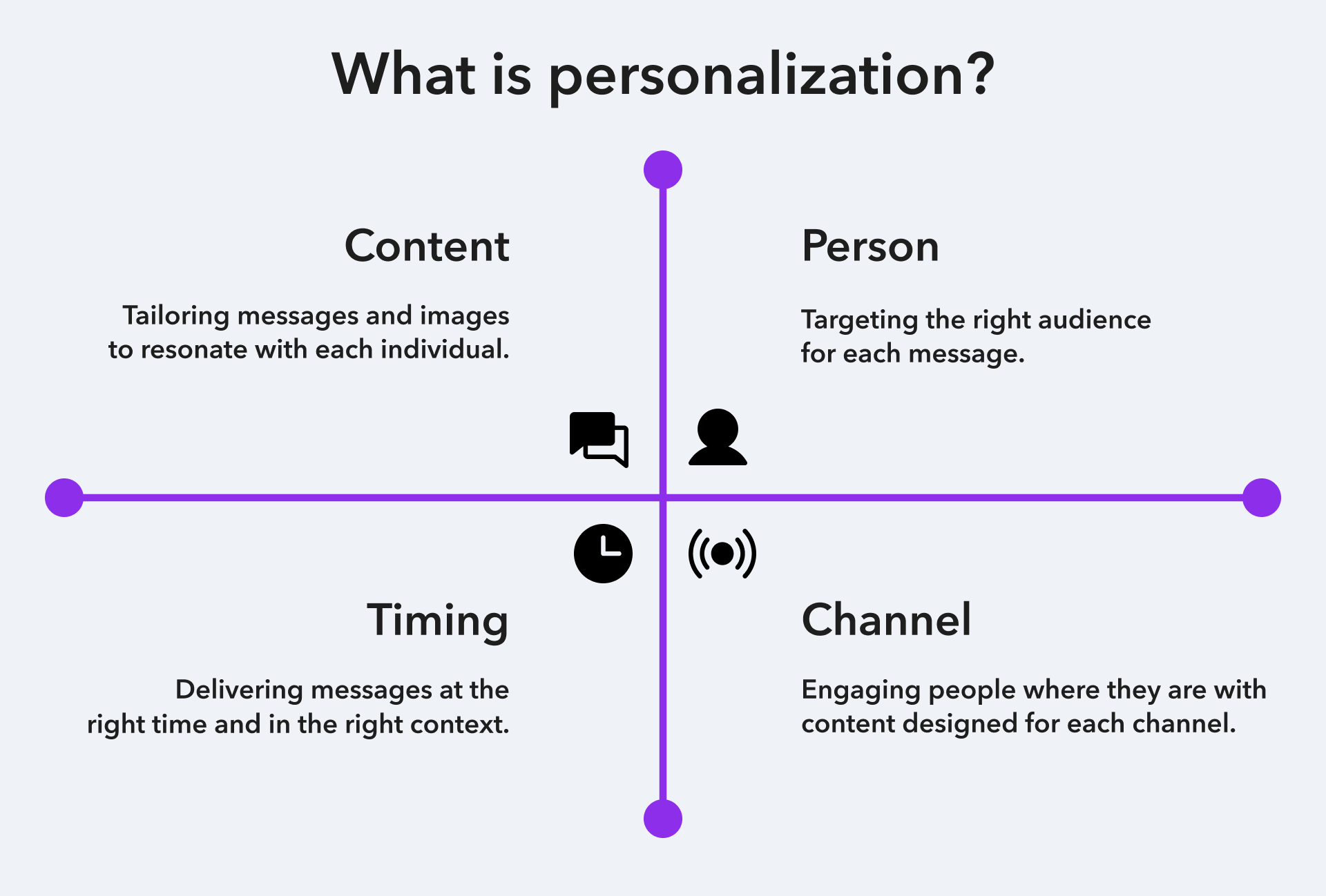
Technology research and consulting firm Gartner defines personalization as “a process that creates a relevant, individualized interaction between two parties designed to enhance the experience of the recipient.”
By anticipating what individuals want and need, businesses can personalize user interactions so that customers feel valued, creating more positive user experiences and profitable customer relationships.
Personalization in practice
Successful personalization feels natural, not creepy or robotic. It’s social media advertisements featuring shirts that look similar to styles you've been browsing online elsewhere, the concerts your music streaming software serves based on your location, or the articles a news outlet recommends reading next based on those you've already perused while sipping your morning coffee.
To provide natural-feeling personalized content, brands must consider not only what customers want but also their customer persona, where they are in the buyer's journey, and what digital channels, devices, and touchpoints they frequent.
When brands get these right, personalization feels authentic and is more likely to win over new customers and retain existing customers.
Personalization versus customization
Customization and personalization are often used interchangeably, but they are different things.
The critical difference is that customization requires the customer to take action, while personalization is implemented as part of the brand experience.
Customization describes a customer's ability to change or modify their experience or a product. It gives them some control over how they interact with your brand and allows them to directly shape their experience.
For example, you can pick a theme for your browser that suits your mood, set ringtones on your phone so you know who’s calling, or adjust the notification settings on your favorite app.
In these examples, your experience is customized, but may not be personalized.
Personalization is how brands stand out
Personalization is how you cut through the noise of impersonal ads and connect one-on-one with customers. It elevates the customer experience with personalized marketing that resonates with individual pain points and desires and directs users to their personal best solution.
Successful personalization turns casual visitors into loyal customers. Brands that provide personalized content see a measurable impact on click-through rate (CTR) and conversions.
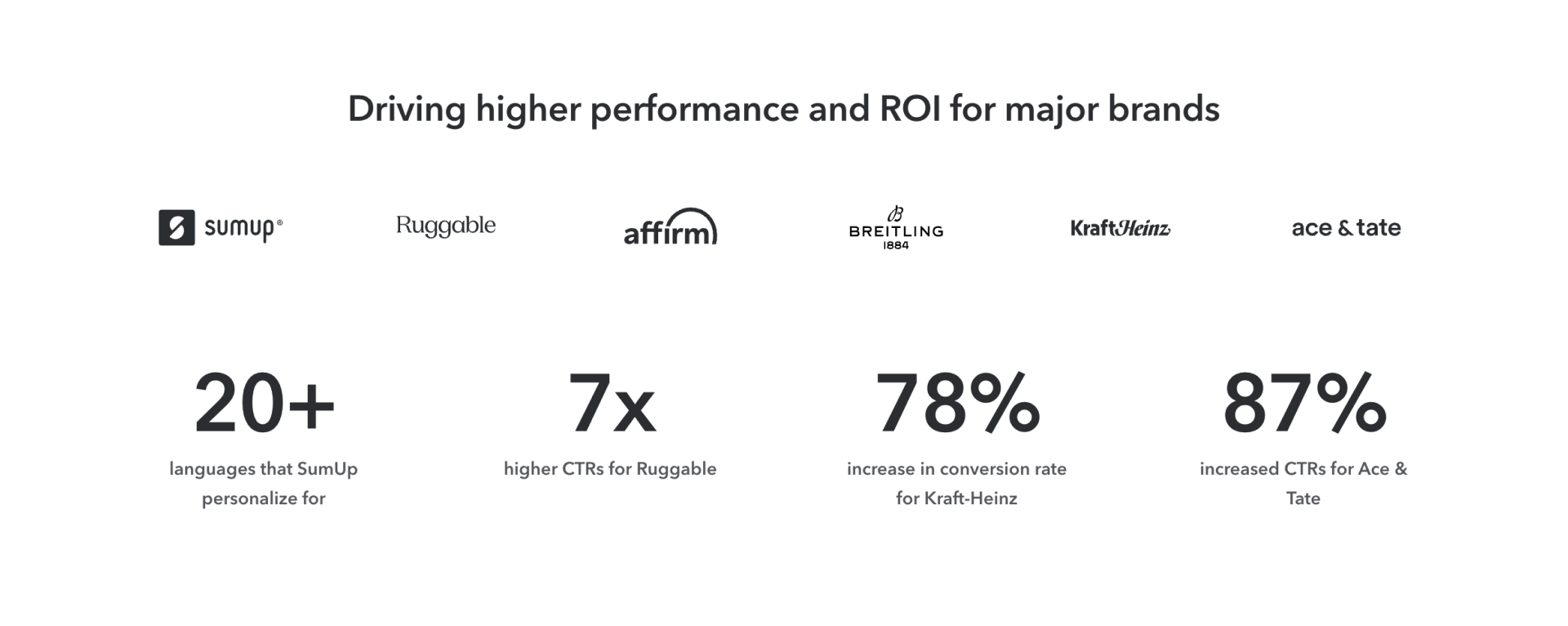
Personalization and experimentation
Personalization and experimentation go hand in hand in helping brands deliver a superior customer experience.
Experimentation helps companies understand what works best for their audiences. It's how you identify the right combination of content, audience, channel, and timing to personalize interactions successfully.
Experimentation should be part of any personalization strategy. As personalization becomes more widespread and integrated into our lives, it will become increasingly important for companies across industries to get personalization right.
That means using experimentation to understand customer behavior and what users truly want instead of relying on guesswork.
See how Ace & Tate uses A/B experiments to improve click-through rates.
What are the different methods of personalization?
When personalization entered the digital marketing scene, a single method existed: rules-based personalization. As purpose-built software became available, this method of personalization became easier to manage, and a new method of personalization, algorithmic/machine learning, emerged.
Below, we provide an overview of each approach, including its pros and cons. Then, we explore how the two are sometimes combined to create a third personalization category, hybrid personalization.
Rules-based personalization
Rule-based personalization takes an if/then approach to personalizing experiences. This type of conditional logic means that “if” a customer performs an action or meets certain criteria, “then” they will receive a specific type of content.
In this approach to personalization, brands begin by manually dividing customers into well-defined segments. Then, they match those segments to specific products, content, or experiences based on the group they fit into or the attributes they possess.
A/B testing plays an important role here as initial segments and conditions are based on assumptions that need to be continuously tested and iterated on.
Rules-based personalization can be as simple as bucketing customers into three categories such as new customers, returning customers, and past customers. Or, it can get more granular, with brands including a customer's location, device, or other data points in the segmentation.
The more granular the efforts are, the more time and testing will be needed to effectively tailor conditional statements.
For this reason, the most effective personalization deals with simple variables.
Added values and drawbacks of rules-based personalization
Added value | Drawback |
---|---|
Easy-to-adjust content – Because segments and content are manually set up and linked, teams can track performance metrics and easily adjust content and segmentation to improve program optimization. | Difficult to add new customer segments – Each time customer personas change or expand, content and conditional statements must be manually added, adjusted, and assigned in alignment. Content creators might struggle to keep up with these tasks as you grow and scale. |
Straightforward – With rules-based personalization, customer segments, the content being tailored, and the governing rules are relatively clear. This clarity makes them easy to share with stakeholders. | Constricts customers – Not everyone fits neatly into a single customer segment — those driving the personalization effort or the software must decide which group is the closest fit. In these instances, the content a customer receives might not resonate with them. |
Affordable – This approach skips expensive software, meaning less financial investment up front. The trade-off is the amount of time your team will have to invest. Rules-based personalization demands extensive critical thinking during setup and constant customer segment management over time — the financial implications of which can be seen in payroll. | Time-consuming to set up – This type of personalization requires planning and strategy that's best informed by cross-functional stakeholders. Getting sales, marketing, engineering, and customer service teams to fully align on the strategy can delay your launch date big time. |
Algorithmic/machine learning personalization
Algorithmic personalization, machine learning personalization, and predictive personalization all describe a similar approach to personalization, which relies on machine learning and data-driven algorithms to deliver data-informed content to customers.
Personalization engines and data analytic tools work together to identify individual customer needs and then make real-time calculations to deliver the most appropriate content.
This one-to-one personalization method relies heavily on automation, data, and technology.
There are several standard machine learning personalization models that businesses can choose to implement — they may even decide to pair several in support of highly targeted experiences.
Basic models use sequence detection to predict what customers might want or do next based on previous behaviors, patterns, or characteristic classifications that act as key personal identifiers.
More advanced models track an array of signals to consistently "learn" about customers and serve tailored experiences that aim to attract attention now, and then maintain it into the future, maximizing customer lifetime value.
With these more advanced models, every action a customer takes or doesn't take informs how the personalization engine will serve that individual and other customers in the future.
Added values and drawbacks of algorithmic personalization
Added value | Drawback |
---|---|
Advanced targeting – With access to diverse, high-quality data, experiences can be tailored to individual customers over large and finite customer segments, expanding the types of products, services, and experiences being served. | Expensive – While the ROI can be rich if done successfully (for example, Netflix reduces churn by $1 billion dollars annually with its personalized recommendations), the tools necessary to carry out machine learning personalization can quickly eat up budgets. |
Less maintenance, more scaling – Once personalization, analytics, and content tools are set up, little maintenance is required. Unlike rules-based personalization, algorithmic personalization recognizes, indexes, and tailors experiences for customers when they enter the site, with no manual segmentation needed. | Data reliant – With so much reliance on accurate, high-volume, and regularly updated customer information, machine learning personalization is at the mercy of growing data and user privacy laws. Europe's General Data Privacy Act and California's Consumer Privacy Act are only a sample of what's to come. |
A/B testing isn't winner-takes-all – When running A/B tests with algorithmic personalization, you can determine which variations work best for different customer segments and individuals rather than deploying one winning variation to everyone. | Potentially intrusive – Customers want to feel seen and heard — within reason and on their terms. With massive amounts of data to base one-of-a-kind experiences on, machine learning can sometimes cross the line between caring and creepy. |
Hybrid personalization
Many of the drawbacks of rules-based personalization are offset by the benefits of an algorithmic approach, and vice versa.
Hybrid personalization recognizes the balance that these two can create and combines certain aspects of each to deliver individually tailored content that also considers what customer segment someone is likely to fit into and whether that should override any content being delivered via algorithms.
With hybrid personalization, brands pair business rules with algorithmic personalization, which makes for more intentional, less invasive experiences.
This curated approach to personalization also lets companies build out personalization motions that might suit specific campaigns or business needs.
For example, Apple might use hybrid personalization to recommend its new iPhone to a customer who bought a MacBook on a mobile device. This rule would override the company's go-to algorithmic recommendation which might suggest that the customer purchase something to go with their new computer, say a mouse or keyboard, based on sequential buying patterns seen in similar customers.
In setting up this hybrid personalization rule, Apple can promote its new iPhone to more individuals temporarily without affecting algorithmic suggestions that exist elsewhere in the customer experience.
When launching personalization efforts for the first time, businesses may need guidance on which approach to select. Smaller businesses might be financially positioned to implement a rules-based approach and then, over time, migrate to a hybrid or algorithmic approach as they're able to make more sizable technology investments and have greater customer data to pull from.
See how AI-native personalization helps marketing teams of all sizes deliver personalized experiences at scale.
Customer data fuels personalization
Customer data is the foundation of any personalization strategy. It’s what allows you to make relevant associations and deliver personalized experiences. Managing customer data effectively and efficiently is critical to creating personalized customer experiences.
Types of data used for personalization
We can categorize data into four types based on collection methods:
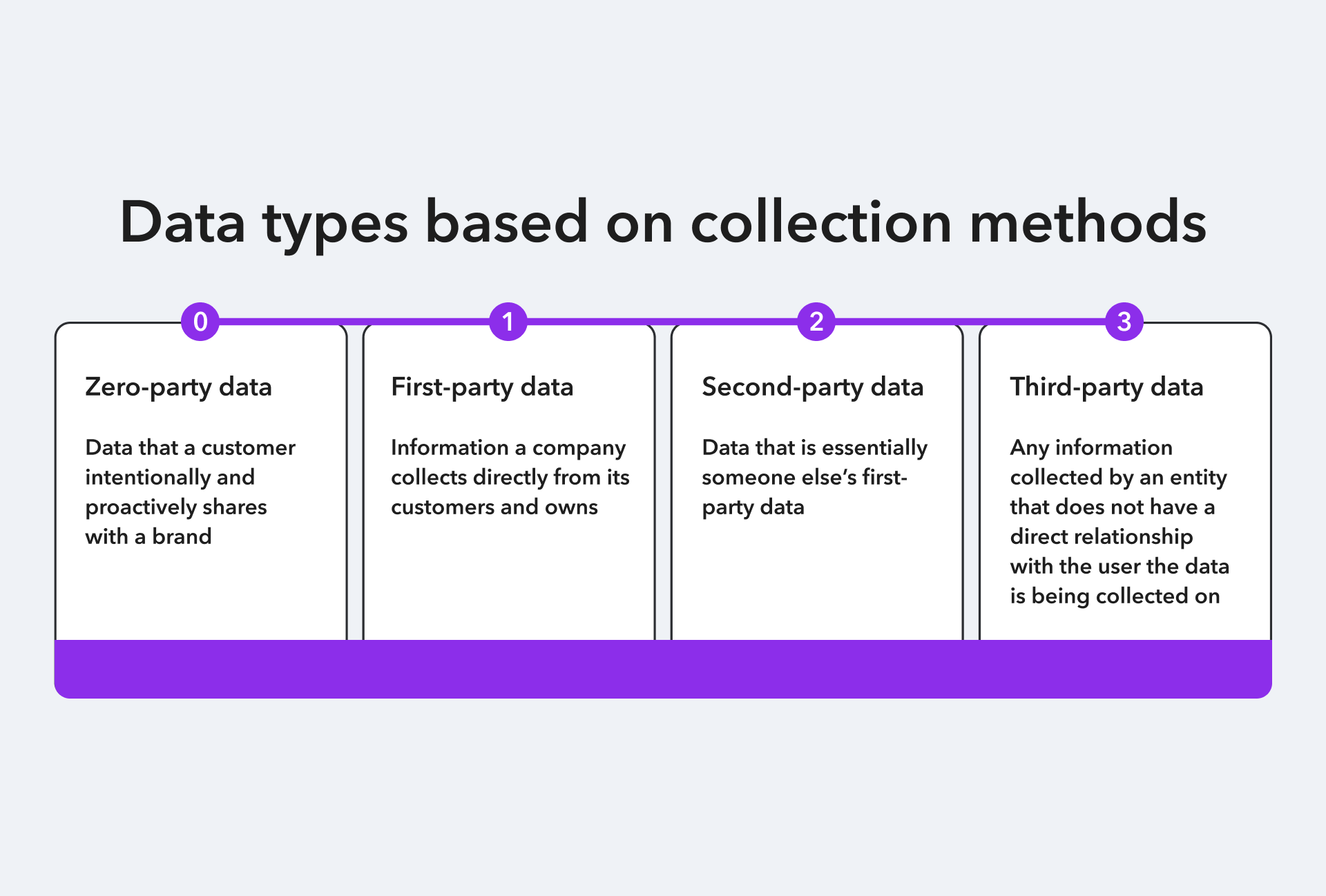
Zero-party data:
Coined by Forrester Research, zero-party data is defined as “data that a customer intentionally and proactively shares with a brand, which can include preference center data, purchase intentions, personal context, and how the individual wants the brand to recognize her.”
Zero-party data is valuable because it's not biased and does not violate the user's privacy since it is collected with a user's permission and not by inference.
First-party data:
First-party data is information about a customer collected directly by a company through its own channels and sources. It is a firm's own data that they do not have to buy or sell to third parties since the client has already willingly provided it throughout their interactions with the company.
However, when acquired without the user's express consent, first-party data raises privacy problems.
Companies are obligated by law to get consumer permission before collecting personal information, although these requirements are subject to national rules such as the GDPR and the CCPA.
Second-party data:
Second-party data is information that you didn't get yourself. It is essentially someone else’s first-party data. It's sometimes applied between trustworthy companies who agree to share audience data if it's valuable to both of their enterprises, but it raises more privacy concerns than zero-party or first-party data.
Third-party data:
Third-party data is information gathered from several sources, consolidated into a single dataset, packaged, and sold. Companies that sell third-party data usually collect first-party data from a range of different businesses and bundle it for sale.
Third-party data has been criticized in recent years, and as a result, Apple and Google have both taken steps against it, with Apple blocking third-party cookies and Google removing the support of third-party cookies from Chrome.
Breaking customer data down further
In addition to categorizing data by collection methods, you can further organize your data to better understand the information you have.
For example, in the following chart, we’ve organized data into demographic data, geographic data, behavioral data, psychographic data, firmographic data, and technographic data.
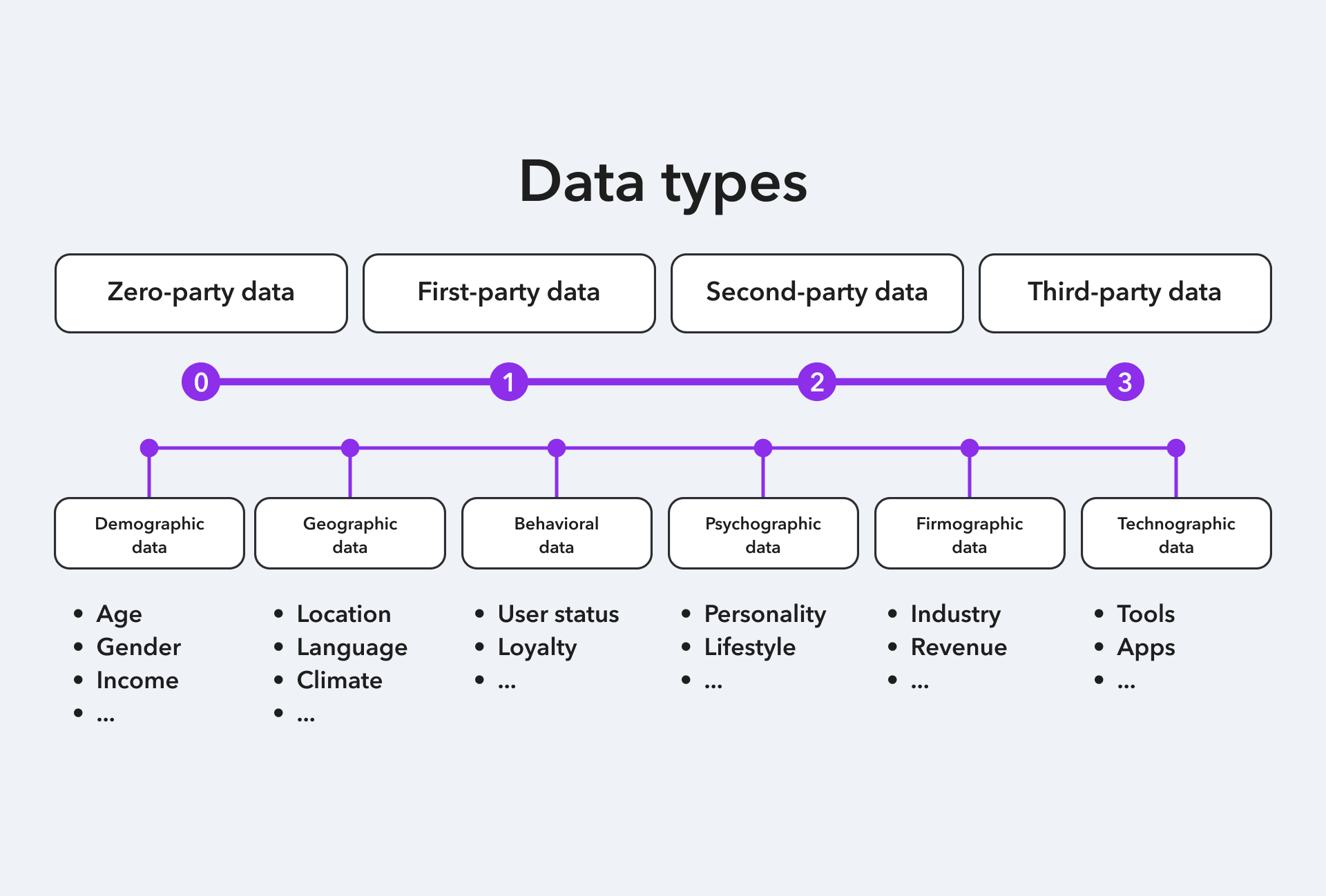
Common personalization challenges (and how to overcome them)
Successful personalization drives increased revenue and market share by providing the right products at the right time to the right customers.
Some companies struggle to get personalization right, resulting in operational disruption, increased costs, and reputational damage.
Understanding common personalization challenges and how to avoid them will help you build a more effective personalization program.
Common personalization challenges include
Privacy concerns:
Privacy concerns related to personalization are mostly about how businesses collect customer data. Getting consent, inviting customers to share information intentionally, using first-party data, and being transparent about data use can help mitigate these concerns.
Content management and scalability:
One of the most significant challenges to achieving personalization at scale is the time and effort required to create and manage all the versions of content needed for each audience segment. Choosing an AI-native personalization platform that integrates with your content management solution can significantly reduce this burden, enabling even small teams to launch sustainable personalization programs.
Data collection and incomplete customer profiles:
We’ve all heard the adage, “garbage in, garbage out.” Personalization relies on data to produce the most relevant content. If you don't collect enough data or if there's too much noise within what you've collected (i.e., irrelevant data), then personalized content can miss the mark. Your current tech stack needs to work seamlessly, pulling data from diverse sources to create robust, targeted profiles so you can deliver the best possible personalized experience for your users.
Lack of organizational knowledge and capabilities:
Personalization at scale is a tall order, and customers can be unforgiving if you get it wrong: 57% of customers say they would switch to a company’s competitor due to one bad customer experience. Look for tech partners who are experts in personalization and offer enterprise-level support to get you started. Good partners can help you avoid common missteps and fill in the gaps while you build personalization capabilities.
See how Contentful helps customers deliver personalization at scale.
How to get started with personalization
With personalization aiding in customer satisfaction, conversion, and retention, developing a sustainable personalization strategy is at the top of many to-do lists.
Before you get started, it’s helpful to think through the following components of the personalization process:
-
Which customer segments will you target? A common mistake is to get too granular too soon. Understanding the personalization maturity journey can help companies build progressively more sophisticated personalization capabilities.
-
What content are you tailoring? Again, starting small and expanding your personalization strategy is the key to long-term success.
-
Which type of personalization will you use? Rules-based, algorithmic, or hybrid?
-
What data will you use to power your personalization strategy? Think about how you will integrate data directly into the personalization process.
-
How will you measure the effectiveness of your personalization efforts? Experimentation is the key to continually improving personalization to deliver the best customer experiences possible.
Understanding where your business stands in each of these areas will help you choose the right personalization strategy and the technologies you need to support it.
Ready to ramp up your personalization efforts? Contentful can help you start fast and scale smoothly. Schedule time to chat with our team about your personalization goals.
Up next: Content personalization
Learn the benefits of website content personalization, key types, and where to apply it, from headlines and CTAs to navigation and featured content.
Written by
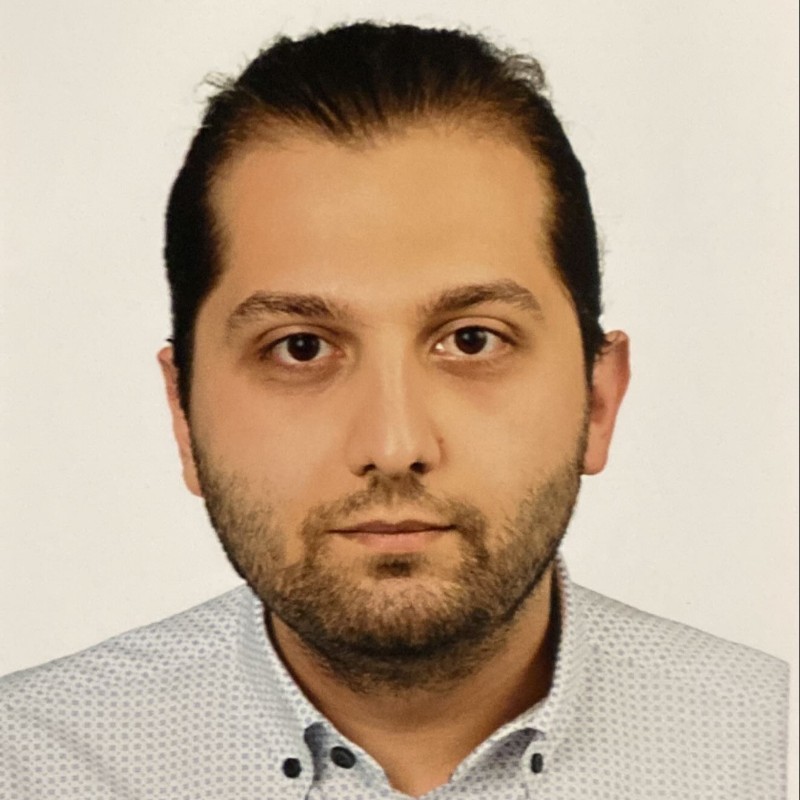
Esat Artug
Esat is Product Marketing Manager at Contentful and sharing his thoughts about personalization, digital experience, and composable across various channels.